Unveiling the Path to AI Fairness: Tackling Bias for a More Equitable Future
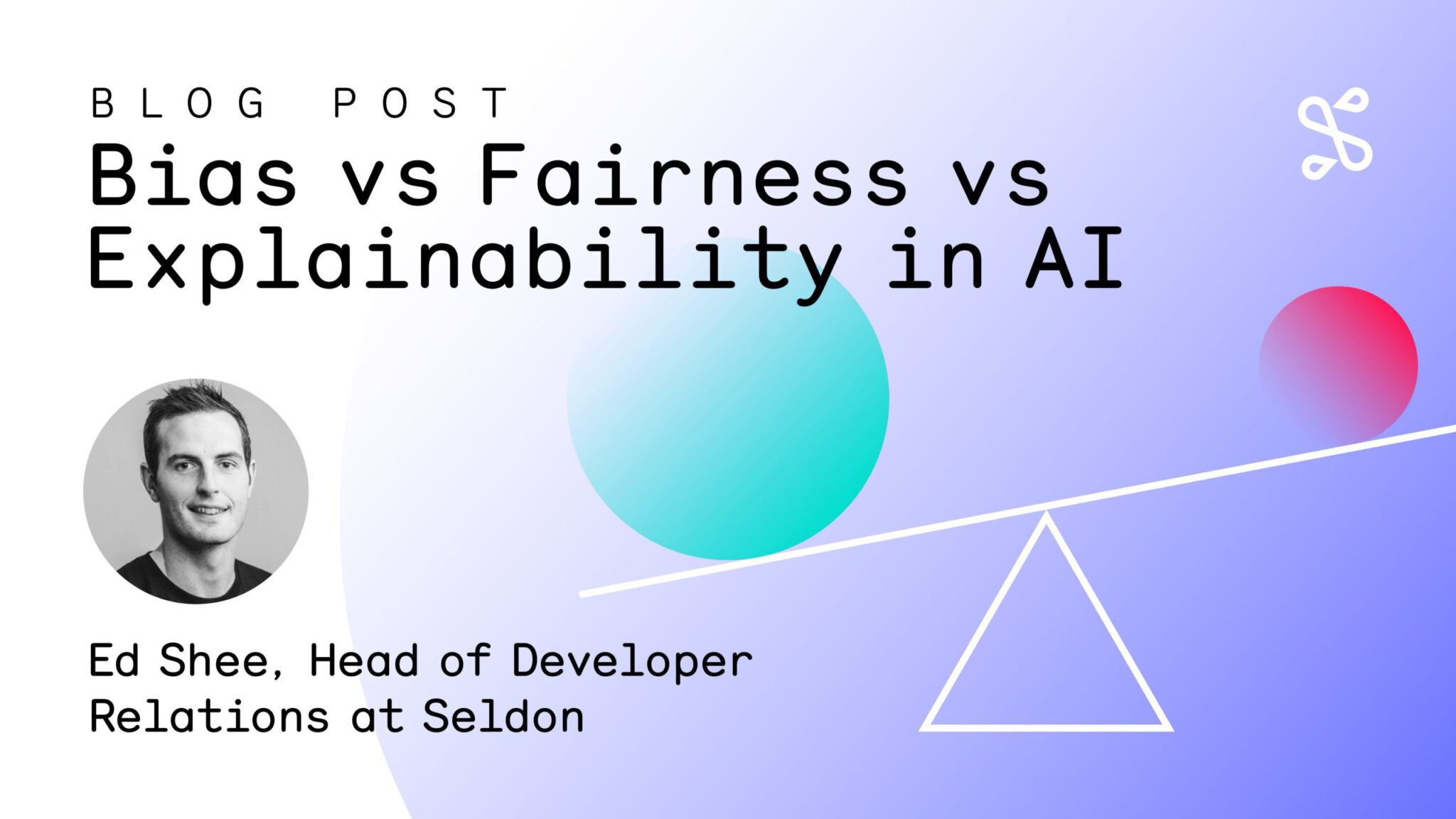
Welcome to our enlightening blog post on the captivating topic of AI Bias and Fairness. In today's rapidly evolving world, artificial intelligence has become an integral part of our daily lives, shaping various aspects of society. However, as AI becomes more prevalent, it is crucial to address the issue of bias and ensure fairness in its implementation. Join us on this thought-provoking journey as we explore the intricacies of AI bias, uncover its potential consequences, and discover the steps we can take towards a future that embraces unbiased and equitable AI solutions.
Section 1: Understanding AI Bias: Delving into the Complexities
Artificial Intelligence (AI) has revolutionized various industries, from healthcare to finance, and has the potential to transform society for the better. However, it is important to acknowledge that AI systems are not immune to bias, as they are designed and trained by humans who can inadvertently introduce their own biases into the algorithms.
AI bias refers to the systematic and unfair favoritism or discrimination displayed by AI systems towards certain individuals or groups. It can result in unequal treatment, perpetuation of stereotypes, and exclusion of marginalized communities. Understanding the complexities of AI bias is crucial in order to address and rectify its impact on society.
The Roots of AI Bias
AI bias can arise from various sources. One primary factor is biased training data, which occurs when the data used to train AI systems is unrepresentative or contains inherent biases. For example, if an AI algorithm is trained on historical data that reflects discriminatory practices, it may learn to perpetuate those biases in its decision-making.
Another factor contributing to AI bias is the influence of human biases during the development process. Developers may inadvertently introduce their own biases when designing algorithms or selecting features, which can lead to biased outcomes. These biases can be conscious or unconscious, making it imperative to address the issue at its root.
The Challenges of AI Bias
Addressing AI bias is not a straightforward task. It requires interdisciplinary collaboration between technologists, ethicists, policymakers, and various stakeholders. One of the primary challenges is the lack of clear guidelines and standards for assessing and mitigating bias in AI systems. Without defined frameworks, it becomes difficult to hold developers and organizations accountable for the potential harm caused by biased algorithms.
Additionally, AI bias can be particularly challenging to detect and mitigate in complex machine learning models. The inner workings of these models are often opaque, making it hard to identify where and how biases are introduced. This calls for the development of transparent and interpretable AI systems that can be audited for bias.
The Need for Fairness in AI
Fairness in AI is not just an ethical concern; it is a fundamental aspect of creating a just and equitable society. By addressing AI bias, we can ensure that AI systems do not perpetuate or amplify existing societal inequalities. Fairness in AI can lead to improved decision-making, increased trust in technology, and greater inclusivity for all individuals, irrespective of their background or characteristics.
Moreover, fairness in AI has the potential to unlock innovation and drive economic growth. By eliminating bias, AI systems can provide equal opportunities for all, fostering a diverse and thriving ecosystem where everyone can contribute and benefit.
In the upcoming sections, we will delve deeper into the impact of AI bias, explore techniques to overcome bias in machine learning, and discuss the importance of data governance and collaboration in building a future of inclusive and unbiased AI.
Section 2: The Impact of AI Bias: Uncovering Unintended Consequences
AI bias can have profound and far-reaching consequences across various domains, highlighting the urgent need for fairness in AI systems. In this section, we will explore real-world examples that illustrate the potential harm caused by biased AI algorithms.
1. Healthcare Disparities
In the realm of healthcare, AI bias can lead to disparities in diagnoses, treatments, and outcomes. For instance, if an AI system is trained on a dataset that predominantly includes data from certain demographics, it may result in biased healthcare recommendations that neglect the needs of underrepresented groups. This can perpetuate existing healthcare disparities and hinder access to quality care for marginalized communities.
2. Financial Discrimination
AI algorithms used in financial institutions can inadvertently discriminate against individuals based on their race, gender, or socioeconomic background. If historical data used to train these algorithms reflects biased lending practices or discriminatory patterns, it can result in unfair credit scoring or loan approvals. This can further exacerbate existing inequalities and limit financial opportunities for marginalized groups.
3. Criminal Justice System Bias
Biased AI systems can have severe implications within the criminal justice system. For example, if an AI algorithm used for predictive policing is trained on biased historical crime data, it may unfairly target specific neighborhoods or communities, perpetuating racial profiling and unjust surveillance practices. Similarly, biased risk assessment algorithms can lead to harsher sentences or incorrect predictions of recidivism, disproportionately affecting marginalized individuals.
4. Employment Discrimination
AI algorithms used in hiring and recruitment processes can inadvertently perpetuate biases and discrimination. If these algorithms are trained on biased data or learn from biased human decision-making, they can reinforce existing hiring patterns that favor certain demographics over others. This can lead to systemic exclusion, hindering diversity and perpetuating inequality in the workplace.
5. Social Bias Amplification
AI algorithms deployed on social media platforms can unintentionally amplify existing social biases and echo chambers. If these algorithms prioritize content based on user preferences and engagement, they can inadvertently reinforce and promote biased or extremist viewpoints. This can have detrimental effects on public discourse, societal cohesion, and the spread of misinformation.
These examples underscore the critical importance of addressing AI bias and striving for fairness in AI systems. In the next sections, we will explore techniques and approaches to overcome bias in machine learning, as well as the role of data governance and collaboration in building a future that embraces unbiased AI solutions.
Section 3: Unmasking the Sources of Bias: Human Influence and Data Imbalance
AI bias can emanate from various sources, including biased training data and the influence of human biases during the development process. In this section, we will delve into these sources and shed light on their contribution to the perpetuation of AI bias.
1. Biased Training Data
One significant source of AI bias is biased training data. AI systems learn from the data they are trained on, and if the data is unrepresentative or contains inherent biases, the resulting algorithms can perpetuate and even amplify those biases.
For example, if an AI algorithm is trained on historical data that reflects discriminatory practices or societal biases, it may learn to replicate those biases in its decision-making. This can lead to unfair treatment or exclusion of certain individuals or groups.
2. Lack of Data Diversity
Data diversity plays a crucial role in mitigating AI bias. If the training data is not diverse enough, it can lead to skewed representations and inadequate coverage of certain demographics or characteristics.
For instance, if a facial recognition algorithm is predominantly trained on data that represents lighter-skinned individuals, it may struggle to accurately recognize or categorize individuals with darker skin tones. This can result in biased outcomes and discriminatory practices.
3. Human Biases in Algorithm Development
Another source of AI bias is the influence of human biases during the development process. Developers, consciously or unconsciously, can introduce their own biases when designing algorithms, selecting features, or setting decision thresholds.
These biases can stem from societal norms, cultural beliefs, or personal experiences. If left unchecked, they can manifest in biased outcomes and perpetuate discrimination or unfair treatment in AI systems.
4. Lack of Ethical Guidelines
The absence of clear ethical guidelines and standards for addressing AI bias can also contribute to its perpetuation. Without explicit guidelines, developers may not be aware of the potential bias in their algorithms or may lack the necessary tools and frameworks to mitigate bias effectively.
Establishing robust ethical guidelines and standards that promote fairness and inclusivity is crucial in creating AI systems that prioritize unbiased decision-making and mitigate the impact of human biases.
By unmasking the sources of bias and understanding their impact, we can take proactive measures to address and rectify AI bias. In the upcoming sections, we will explore techniques and approaches to overcome bias in machine learning and discuss the significance of algorithmic transparency and data governance in promoting fairness in AI systems.
Section 4: The Ethical Imperative of Fairness: Promoting Social Justice
Fairness in AI is not just a technological concern; it is an ethical imperative that upholds the principles of social justice and equality. In this section, we will explore the interconnectedness between fairness, social justice, and AI, emphasizing the importance of designing AI systems that align with fundamental human rights and values.
1. Reducing Discrimination and Bias
Fairness in AI is essential for reducing discrimination and bias in decision-making processes. By addressing AI bias, we can strive towards a society where individuals are treated fairly and equitably, regardless of their race, gender, age, or any other characteristic.
AI systems that are designed with fairness in mind can help mitigate the perpetuation of societal biases and contribute to a more inclusive and just society.
2. Equal Opportunities and Access
Fairness in AI is pivotal for providing equal opportunities and access to resources and services. AI systems that are biased can reinforce existing disparities and limit opportunities for marginalized communities.
By embracing fairness in AI, we can ensure that everyone has an equal chance to benefit from technological advancements, education, healthcare, and other essential services.
3. Challenging Power Imbalances
AI systems have the potential to challenge power imbalances and disrupt systemic inequalities. However, biased AI can reinforce existing power structures and perpetuate injustice.
By incorporating fairness into AI systems, we can challenge these power imbalances and foster a more equitable distribution of opportunities, resources, and decision-making power.
4. Ethical Responsibility and Accountability
Developers, policymakers, and organizations bear an ethical responsibility to ensure that AI systems are fair and just. They must be held accountable for the potential harm caused by biased algorithms and discriminatory practices.
By prioritizing fairness and incorporating ethical considerations into AI development and deployment, we can build trust in AI technologies and foster a responsible and accountable AI ecosystem.
Ultimately, fairness in AI is not just a technical challenge; it is a moral imperative. By striving for fairness and aligning AI systems with social justice principles, we can create a future where AI serves as a powerful tool for positive change, devoid of bias and discrimination.
Section 5: Unbiased AI: Striving for Algorithmic Transparency
Transparency is a crucial aspect of achieving unbiased AI systems. In this section, we will explore the significance of algorithmic transparency in combating AI bias, the methods for auditing AI systems, and the importance of involving diverse voices in the development process.
1. The Importance of Algorithmic Transparency
Algorithmic transparency refers to the ability to understand and interpret how AI systems make decisions. Transparent AI algorithms provide clear explanations for their outputs, allowing users and stakeholders to comprehend the reasoning behind the decisions made.
By ensuring algorithmic transparency, we can identify and address any biases or discriminatory patterns that may arise in AI systems, promoting fairness and accountability.
2. Auditing AI Systems for Bias
Auditing AI systems is essential for identifying and mitigating bias. Audits involve examining the training data, algorithms, and decision-making processes to uncover any potential biases or unfair outcomes.
Through rigorous auditing, we can detect and rectify biases, ensuring that AI systems are fair, equitable, and aligned with ethical standards.
3. Involving Diverse Voices
Ensuring diverse representation and perspectives in the development of AI systems is crucial for addressing bias and promoting fairness. By involving individuals from diverse backgrounds, we can minimize the risk of introducing unconscious biases and ensure that the AI systems are designed to be inclusive and unbiased.
By incorporating diverse voices, we can foster innovation and create AI systems that are more attuned to the needs and experiences of different communities.
4. Ethical Considerations in Design
Designing AI systems with ethical considerations at the forefront can help prevent and mitigate bias. This involves being conscious of the potential societal impact of AI algorithms and making intentional decisions to prioritize fairness and inclusivity.
By embedding ethical considerations into the design process, we can create AI systems that align with our values and contribute to a more equitable and just society.
By striving for algorithmic transparency, conducting thorough audits, involving diverse voices, and incorporating ethical considerations into AI design, we can take significant steps towards building unbiased AI systems. In the upcoming sections, we will explore techniques to overcome bias in machine learning and discuss the role of data governance in promoting fairness in AI.
Section 6: Overcoming Bias in Machine Learning: Techniques and Approaches
Machine learning techniques play a vital role in combating AI bias. In this section, we will introduce various approaches, such as debiasing algorithms, fairness-aware learning, and post-processing techniques, that can be employed to reduce or eliminate bias in AI systems.
1. Debiasing Algorithms
Debiasing algorithms aim to mitigate bias by adjusting the training data or modifying the learning process. Techniques such as reweighing, which assigns different weights to different data samples, or resampling, which balances the training data, can help reduce bias and promote fairness in AI systems.
By debiasing algorithms, we can address the disparities and discrimination that may arise from biased training data, leading to more equitable decision-making.
2. Fairness-Aware Learning
Fairness-aware learning approaches explicitly incorporate fairness constraints into the learning process. These techniques involve optimizing the AI models not only for accuracy but also for fairness metrics.
By incorporating fairness as a core objective, fairness-aware learning techniques can help prevent and mitigate bias, ensuring that AI systems make fair and unbiased decisions.
3. Post-Processing Techniques
Post-processing techniques involve modifying the outputs of AI systems to ensure fairness. These techniques include methods such as threshold adjustment, where decision thresholds are adjusted to achieve fairness, or equalized odds, where the error rates are balanced across different demographic groups.
By applying post-processing techniques, we can correct any biases that may exist in the predictions and outputs of AI systems, promoting fairness and equal treatment for all individuals.
4. Continuous Monitoring and Evaluation
Overcoming bias in machine learning is an ongoing process that requires continuous monitoring and evaluation. It is crucial to regularly assess the performance of AI systems, examine the impact on different groups, and identify any emerging biases.
By continuously monitoring and evaluating AI systems, we can proactively identify and rectify biases, ensuring that the systems remain fair and unbiased over time.
By employing techniques such as debiasing algorithms, fairness-aware learning, post-processing, and continuous monitoring, we can make significant progress in overcoming bias in machine learning. In the upcoming sections, we will discuss the role of data governance in promoting fairness in AI and explore the significance of collaboration in building unbiased AI systems.
Section 7: The Role of Data Governance: Ensuring Data Quality and Diversity
Data governance plays a crucial role in combating AI bias and promoting fairness in AI systems. In this section, we will explore strategies for ensuring data quality, promoting diversity in training datasets, and implementing robust data governance frameworks that foster fairness and prevent bias propagation.
1. Ensuring Data Quality
Data quality is essential for building unbiased AI systems. It is important to ensure that the training data used for AI algorithms is accurate, reliable, and representative of the real-world population.
By implementing rigorous data collection processes, data cleaning techniques, and quality assurance measures, we can minimize the risk of introducing biases through faulty or incomplete data.
2. Promoting Diversity in Training Datasets
Diversity in training datasets is vital for reducing bias and ensuring fair AI systems. It is important to include data samples that represent various demographics, socioeconomic backgrounds, and cultural contexts.
By promoting diversity in training datasets, we can mitigate the risk of perpetuating biases and ensure that AI systems are designed to cater to the needs and experiences of a diverse range of individuals.
3. Ethical Data Collection Practices
Ethical data collection practices are essential for data governance and fairness in AI. It is crucial to obtain consent, protect privacy, and adhere to ethical guidelines when collecting and using data.
By prioritizing ethical data collection practices, we can ensure that individuals' rights are respected, and their data is used in a responsible and fair manner.
4. Auditing and Bias Detection
Regular auditing and bias detection processes are integral to data governance. By conducting audits, we can assess the quality and fairness of the data used in AI systems, detect any potential biases, and take corrective actions.
By implementing robust auditing and bias detection mechanisms, we can identify and rectify biases at the data level, ensuring that AI systems are built on a foundation of fairness and integrity.
By prioritizing data governance, ensuring data quality and diversity, promoting ethical data collection practices, and implementing robust auditing and bias detection processes, we can foster fairness in AI systems. In the upcoming sections, we will delve into fairness metrics and evaluation methods and discuss the importance of collaboration in building unbiased AI systems.
Section 8: Fairness Metrics and Evaluation: Measuring Bias in AI Systems
Measuring and evaluating fairness in AI systems is crucial for identifying and addressing bias. In this section, we will delve into different fairness metrics and evaluation methods that can be used to assess and quantify bias, enabling us to make informed decisions and improvements.
1. Fairness Metrics
Fairness metrics provide quantitative measures to assess the fairness of AI systems. These metrics evaluate the extent to which different groups are treated fairly and equitably.
Some common fairness metrics include disparate impact, equal opportunity, and predictive parity. These metrics allow us to identify any disparities or biases in the outcomes produced by AI systems.
2. Evaluation Methods
Various evaluation methods can be employed to assess bias in AI systems. These methods involve testing the performance of AI algorithms on different groups and evaluating the fairness of their outcomes.
Methods such as subgroup analysis, cross-validation, and A/B testing can help uncover any potential biases and provide insights into the performance of AI systems across different demographics or characteristics.
3. Bias Mitigation Strategies
Once bias is identified and quantified, it is crucial to implement bias mitigation strategies. These strategies involve adjusting the AI algorithms, training data, or decision-making processes to reduce or eliminate the identified biases.
By employing bias mitigation techniques, we can work towards creating AI systems that are fair, equitable, and unbiased in their decision-making processes and outcomes.
4. Iterative Improvements
Measuring and evaluating bias in AI systems is an iterative process. It requires continuous monitoring, evaluation, and improvements to ensure that fairness is upheld throughout the development and deployment of AI systems.
By embracing an iterative approach, we can learn from the feedback and insights gained through evaluation, make necessary adjustments, and strive towards continuously improving the fairness and performance of AI systems.
By utilizing fairness metrics, evaluation methods, implementing bias mitigation strategies, and embracing iterative improvements, we can measure and address bias in AI systems. In the upcoming sections, we will discuss the ethical considerations in AI design and the significance of collaboration in building unbiased AI systems.
Section 9: Building a Future of Inclusive AI: Collaboration and Responsibility
Creating unbiased and fair AI systems requires collective effort and a sense of responsibility. In this section, we will emphasize the importance of collaboration among researchers, policymakers, and industries to tackle bias and discuss the ethical responsibility of all stakeholders in shaping an inclusive AI future.
1. Interdisciplinary Collaboration
Addressing bias in AI systems necessitates interdisciplinary collaboration. Researchers, policymakers, ethicists, and industry experts must come together to share insights, knowledge, and best practices.
Through collaboration, we can leverage diverse perspectives and expertise to develop robust solutions that promote fairness and inclusivity in AI.
2. Ethical Responsibility
All stakeholders involved in AI development and deployment bear an ethical responsibility to ensure that AI systems are fair, unbiased, and aligned with societal values. This responsibility extends to developers, policymakers, organizations, and individuals who interact with AI systems.
By upholding ethical principles and holding ourselves accountable, we can collectively work towards building AI systems that respect human rights, promote fairness, and contribute positively to society.
3. Inclusive Decision-Making
Inclusive decision-making processes are essential for ensuring that the benefits and risks of AI are fairly distributed. It is crucial to involve diverse voices and perspectives, representing different demographics, cultures, and backgrounds, in the design, development, and deployment of AI systems.
By embracing inclusive decision-making, we can challenge biases, avoid undue concentration of power, and build AI systems that address the needs and aspirations of all individuals and communities.
4. Education and Awareness
Education and awareness play a vital role in building an inclusive AI future. It is important to promote digital literacy, ethics, and awareness about AI bias among individuals, organizations, and society at large.
By fostering a better understanding of AI and its potential biases, we can empower individuals to make informed decisions, demand transparency, and actively participate in shaping the future of AI in a fair and inclusive manner.
Through collaboration, ethical responsibility, inclusive decision-making, and education, we can build a future where AI systems are fair, unbiased, and inclusive. In the final section, we will reflect on the challenges and opportunities in the pursuit of fairness in AI and the vision of a future free from bias and discrimination.
Section 10: Towards a Fairer AI Landscape: Navigating Challenges and Opportunities
As we conclude this enlightening journey, let's reflect on the challenges and opportunities that lie ahead in the pursuit of fairness in AI. By addressing these challenges head-on and seizing opportunities for innovation, we can pave the way for a future where AI serves as a powerful tool for positive change, devoid of bias and discrimination.
1. Overcoming Technological Challenges
Developing unbiased AI systems poses technological challenges that require innovative solutions. Advancements in algorithmic transparency, bias detection, and debiasing techniques are vital in ensuring that AI systems are fair and free from bias.
By investing in research and development, we can overcome these challenges and create AI technologies that are at the forefront of fairness and inclusivity.
2. Addressing Data Limitations
Data limitations can hinder the fairness of AI systems. Biased or incomplete data can perpetuate discrimination and amplify societal inequalities.
By addressing data limitations, ensuring data quality, and promoting diversity in training datasets, we can mitigate the risk of bias and build AI systems that are more representative and equitable.
3. Policy and Regulation
Policy and regulation play a crucial role in shaping the future of AI. Governments and regulatory bodies need to establish guidelines and standards that ensure fairness, transparency, and accountability in AI systems.
By fostering a supportive regulatory environment, we can create incentives for organizations to prioritize fairness in AI and protect individuals from discriminatory practices.
4. Embracing Diversity and Inclusion
Diversity and inclusion are key pillars in building a fairer AI landscape. Embracing diverse perspectives, experiences, and voices can lead to more robust AI systems that consider the needs and values of a broad range of individuals and communities.
By fostering a culture of diversity and inclusion, we can challenge biases, overcome blind spots, and build AI technologies that are fair, unbiased, and aligned with societal values.
As we navigate the challenges and opportunities in the pursuit of fairness in AI, let us remain committed to the vision of a future where AI promotes social justice, equality, and inclusivity. By working together, we can build an AI landscape that empowers individuals, respects diversity, and creates positive impacts on a global scale.
In conclusion, the journey to address AI bias and promote fairness is a complex yet imperative one. We have explored the intricacies of AI bias, its potential consequences, and the challenges it poses. However, we have also discovered the techniques, approaches, and strategies that can be employed to overcome bias and build unbiased AI systems.
Fairness in AI is not just a technological concern; it is an ethical imperative that upholds the principles of social justice and equality. It requires collaboration among researchers, policymakers, ethicists, and industries to ensure that AI systems are fair, unbiased, and aligned with fundamental human rights and values.
Transparency, data governance, and algorithmic accountability are essential pillars in the pursuit of fairness. By ensuring algorithmic transparency, auditing AI systems, promoting data quality and diversity, and implementing robust governance frameworks, we can foster fairness in AI and prevent bias propagation.
Building unbiased AI systems also calls for continuous monitoring, evaluation, and improvement. It requires us to embrace an iterative approach, learn from feedback, and make necessary adjustments to mitigate bias and ensure fairness throughout the AI lifecycle.
As we navigate the challenges and seize the opportunities, we envision a future where AI serves as a powerful tool for positive change. A future where AI systems are fair, unbiased, and inclusive, contributing to a society that values diversity, respects human rights, and embraces social justice.
Let us strive together to build this future, where AI technologies empower individuals, break down barriers, and create a world that is fair, equitable, and free from bias and discrimination.
Comments
Post a Comment